why tokenization is important in gpt models?
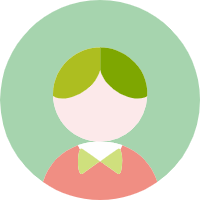
Tokenization is a crucial step in natural language processing (NLP) and artificial intelligence (AI) research, as it serves as the foundation for various tasks and models. In particular, the use of tokenization in generative pre-training Transformer (GPT) models has become a hot topic in recent years. This article will explore the importance of tokenization in GPT models and how it contributes to their performance and efficiency.
1. What is Tokenization?
Tokenization is the process of dividing a text into smaller units called tokens. These tokens can be words, phrases, or characters, depending on the purpose of the tokenization. In NLP and AI, tokenization is often used to break down large text corpora into smaller, manageable datasets for training and testing purposes. This process is essential for ensuring that models can learn useful representations and generalize well across different texts.
2. Why Tokenization Is Important in GPT Models
GPT models, particularly the Generative Pre-training Transformer (GPT) variants, have gained significant attention in recent years due to their remarkable performance on various NLP tasks. These models have proven to be powerful tools for natural language understanding, generation, and other related tasks. However, their success is primarily attributed to the effective tokenization strategies used in their training processes.
a. Enhancing Model Performance
Tokenization plays a crucial role in enhancing the performance of GPT models. By splitting the input text into smaller tokens, the model can better understand and process the text. This allows the model to learn more meaningful representations and generalize better across different text corpora. The smaller token units also make it easier for the model to consume and process the input, leading to improved training efficiency and reduced time-to-solution.
b. Reducing Data Size and Complexity
In large-scale NLP tasks, using tokenization can significantly reduce the size and complexity of the dataset. By breaking down the input text into smaller tokens, the total number of tokens in the dataset is reduced, making it easier for the model to learn and process. This not only speeds up the training process but also reduces the risk of overfitting and improves the generalization ability of the model.
c. Ensuring Consistency and Regularity
Tokenization ensures consistency and regularity in the input data, which is crucial for GPT models. By splitting the input text into smaller token units, the model can more easily identify patterns and regularities in the text, which are essential for successful generalization. Additionally, this consistency also makes it easier for the model to learn transferable representations, allowing it to perform well on various NLP tasks.
3. Conclusion
In conclusion, tokenization is an essential step in the development and training of GPT models. By splitting the input text into smaller tokens, the model can better understand and process the text, leading to improved performance and efficiency. Furthermore, tokenization helps reduce data size and complexity, ensuring consistency and regularity in the input data. As GPT models continue to play a crucial role in various NLP tasks, understanding the importance of tokenization in their training processes is crucial for researchers and practitioners in the field.